The Scan class¶
The pylidc.Scan
class holds some (but not all!) of the DICOM
attributes associated with the CT scans in the LIDC dataset. These attributes
can be used to query the data:
import pylidc as pl
# Query for all CT scans with desired traits.
scans = pl.query(pl.Scan).filter(pl.Scan.slice_thickness <= 1,
pl.Scan.pixel_spacing <= 0.6)
print(scans.count())
# => 31
pid = 'LIDC-IDRI-0078'
scan = pl.query(pl.Scan).filter(pl.Scan.patient_id == pid).first()
Note
Not all of the class attributes are queryable! Some attributes are actually computed properties. You can determine this by, for example, examining the object type for the class:
print(type(pl.Scan.slice_thickness))
# => <class 'sqlalchemy.orm.attributes.InstrumentedAttribute'>
# => ^^^ queryable because it's an sqlalchemy attribute.
# Whereas ...
print(type(pl.Scan.slice_spacing))
# => <type 'property'>
# ^^^ not queryable because it's a computed property
The slice_thickness attribute corresponds to the DICOM attribute
(0018,0050), whereas the slice_spacing attribute is computed from
the median of spacing between the slices
(see pylidc.Scan.slice_zvals()
).
A pylidc.Scan
object has zero or more pylidc.Annotation
objects, which are radiologist annotations of lung nodules found in the scan:
print(len(scan.annotations))
# => 13
Annotation clustering¶
The scan has 13 annotations, but which refer to the same nodule? This can
be determined using the pylidc.Scan.cluster_annotations()
method, which
uses a distance function to create an adjancency graph to determine which
annotations refer to the same nodule in a scan:
nods = scan.cluster_annotations()
print("%s has %d nodules." % (scan, len(nods)))
# => Scan(id=1,patient_id=LIDC-IDRI-0078) has 4 nodules.
for i,nod in enumerate(nods):
print("Nodule %d has %d annotations." % (i+1, len(nods[i])))
# => Nodule 1 has 4 annotations.
# => Nodule 2 has 4 annotations.
# => Nodule 3 has 1 annotations.
# => Nodule 4 has 4 annotations.
Converting scan image values to NumPy array¶
The scan can be converted to a NumPy array for image-processing:
vol = scan.to_volume()
print(vol.shape)
# => (512, 512, 87)
print("%.2f, %.2f" % (vol.mean(), vol.std()))
# => -702.15, 812.52
Scan visualization¶
An interactive GUI can be engaged, with clustered annotations optionally indicated with arrows:
scan.visualize(annotation_groups=nods)
which appears like
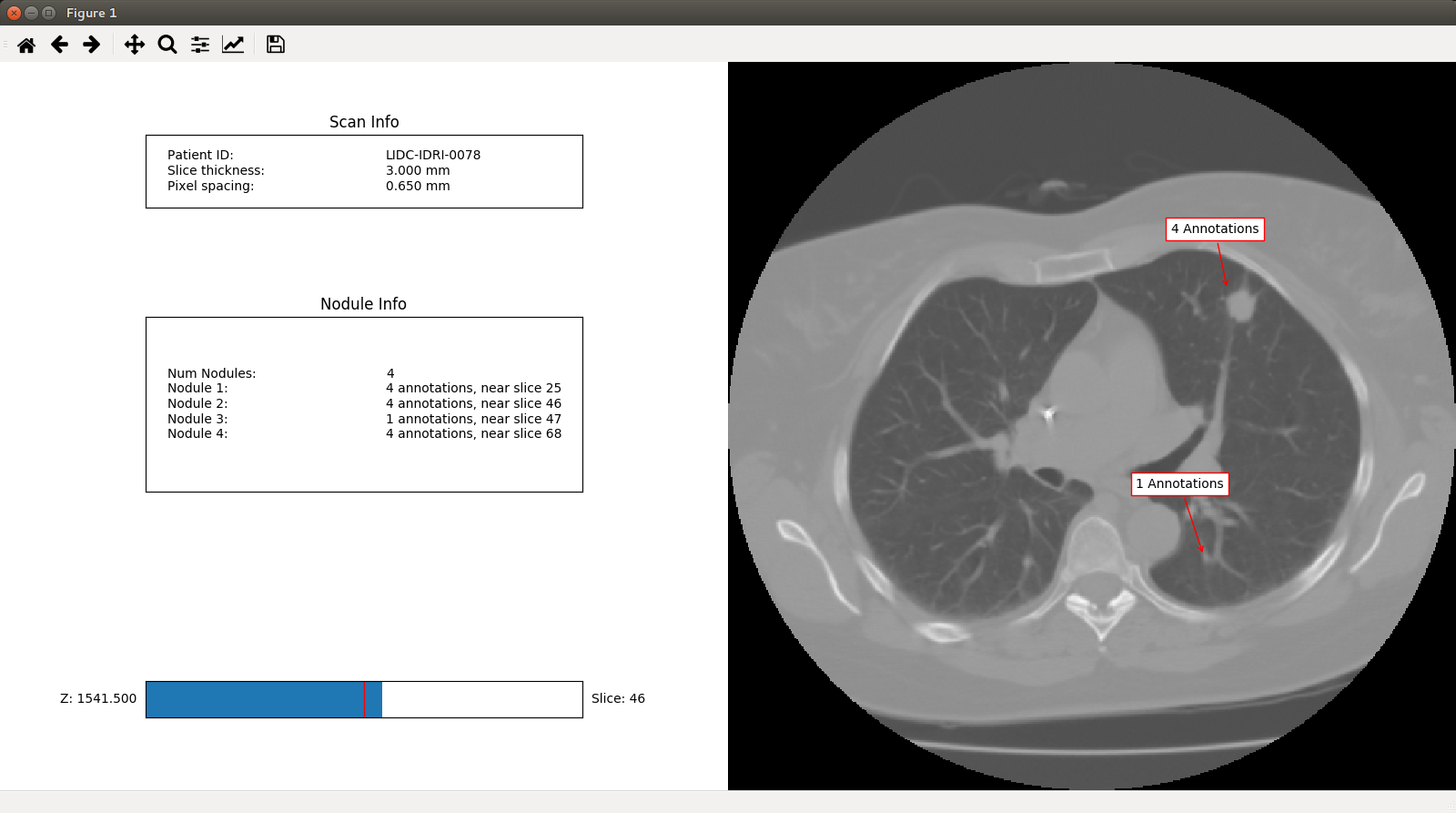